- Yes
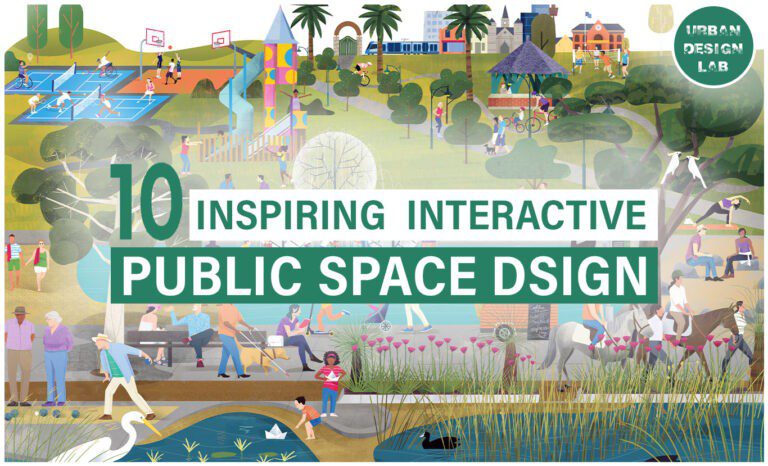
Real-time traffic management solutions, enabled by urban data platforms, provide cities with advanced tools to optimize traffic flow, reduce congestion, and improve safety. These platforms integrate data from various sources—such as sensors, cameras, and GPS—allowing traffic managers to respond to live conditions and adjust signals dynamically. Predictive analytics and AI-driven systems also play a key role, in anticipating congestion and accidents while optimizing routes in real-time. Cities like Singapore, London, and Barcelona have implemented successful real-time traffic management systems, resulting in smoother traffic flow and reduced emissions.
Urban data platforms process vast datasets, turning raw information into actionable insights for traffic managers, while also contributing to sustainability by lowering vehicle idling and pollution levels. However, implementing these systems comes with challenges, such as data privacy concerns, high costs, and the need to integrate with legacy systems. The future of traffic management will see further advancements with autonomous vehicles and smart infrastructure, which will rely heavily on real-time data and predictive technologies to ensure efficient urban mobility and sustainable city development.
Urbanization has dramatically transformed the landscapes of cities worldwide, often leading to environments disconnected from nature. As more people inhabit urban areas, the need to create spaces that promote well-being, sustainability, and ecological balance becomes increasingly important. Biophilic design, which integrates natural elements into urban spaces, offers a solution to these challenges. By reconnecting people with nature through thoughtful design, cities can enhance the quality of life for residents while promoting environmental stewardship. This article explores the principles of biophilic design, its implementation in urban settings, and the benefits it brings to both people and the planet.
Real-time traffic management is the use of live data to monitor and control traffic in a city. Traditionally, traffic management relied on pre-set signals and historical data, but real-time systems use real-time inputs from sensors, cameras, GPS data, and other urban infrastructure to make adjustments on the fly. This allows for immediate responses to traffic incidents, congestion, or unexpected events. According to Alexander (2016), “Real-time traffic management provides dynamic solutions, adjusting to the changing traffic environment moment by moment, thus improving efficiency compared to static systems that cannot adapt in real time”. The use of urban data platforms, which aggregate and analyze massive amounts of information, is key to the success of these systems.
Urban data platforms serve as the backbone of real-time traffic management systems. These platforms integrate data from multiple sources, providing a centralized hub for data collection, processing, and analysis. They utilize advanced technologies such as cloud computing, big data analytics, artificial intelligence (AI), and machine learning to transform raw data into actionable insights.
For example, urban data platforms can analyze patterns in traffic flow, predict congestion hotspots, and recommend optimal signal timings. They can also integrate non-traffic data—such as weather forecasts, special events, or construction schedules—to provide a more holistic view of factors affecting traffic conditions. By delivering these insights to traffic managers, urban data platforms enable smarter decision-making and more efficient use of infrastructure.
Moreover, these platforms facilitate communication between different stakeholders, including traffic authorities, city planners, emergency services, and the public. Real-time information can be disseminated to drivers through mobile apps, variable message signs, and other communication channels, helping commuters make informed choices about their routes and modes of transportation.
Urban data platforms rely on several technological components to function effectively. These include sensors, communication networks, data storage and processing capabilities, and visualization tools.
As Chourabi et al. (2012) note, “The effective implementation of urban data platforms depends on the seamless integration of these components to ensure that the data can be processed, analyzed, and acted upon in real-time”.
Several cities around the world have successfully implemented real-time traffic management systems through urban data platforms.
Singapore
Singapore is renowned for its Intelligent Transport System (ITS), which integrates real-time data to manage traffic efficiently. The Land Transport Authority (LTA) operates an Expressway Monitoring and Advisory System (EMAS) that monitors traffic conditions on expressways using surveillance cameras and incident detection systems. Real-time data allows for quick responses to incidents and dynamic adjustments to traffic signals. The Electronic Road Pricing (ERP) system uses dynamic pricing to manage congestion by charging vehicles entering high-traffic zones during peak hours. These initiatives have helped Singapore maintain smooth traffic flow despite high population density.
London
Transport for London (TfL) utilizes a sophisticated real-time traffic management system to oversee the city’s complex transportation network. By collecting data from over 6,000 traffic signal sites, 1,500 CCTV cameras, and various sensors, TfL adjusts signal timings to optimize traffic flow. The SCOOT (Split Cycle Offset Optimization Technique) system dynamically adjusts signal timings based on real-time traffic demand. TfL also provides real-time traffic information to the public through apps and variable message signs. These efforts have led to a reduction in congestion and improved journey times.
Barcelona
Barcelona has implemented smart city initiatives that include real-time traffic management. The city uses a platform called Sentilo to integrate data from sensors across the urban environment. Traffic data is combined with information on public transport, parking availability, and environmental conditions. By analyzing this data, the city can optimize traffic signals, manage parking resources, and provide real-time information to residents. Barcelona’s efforts have resulted in reduced traffic congestion and enhanced sustainability.
Predictive analytics is a powerful tool in modern traffic management, enabling authorities to anticipate and mitigate congestion before it occurs. By analyzing historical data alongside real-time inputs, predictive models can forecast traffic patterns with a high degree of accuracy.
For example, machine learning algorithms can identify patterns in traffic flow related to time of day, weather conditions, special events, and other variables. These models can predict when and where congestion is likely to occur, allowing traffic managers to implement proactive measures such as adjusting signal timings, deploying traffic officers, or informing the public about potential delays.
In addition, predictive analytics can assist in incident management. By analyzing factors that contribute to accidents or breakdowns, authorities can identify high-risk areas and times, implementing preventative measures such as increased patrols or improved signage.
A study by the U.S. Federal Highway Administration found that predictive analytics could reduce traffic delays by up to 20% in urban areas. By shifting from reactive to proactive traffic management, cities can significantly enhance efficiency and safety.
Artificial intelligence (AI) and machine learning (ML) are revolutionizing real-time traffic management by enabling urban data platforms to analyze complex datasets and make decisions autonomously. AI algorithms can detect patterns in traffic data, predict incidents, and optimize routes in ways that would be impossible for human operators to manage manually. “AI-powered traffic management systems can predict accidents and optimize signal timings based on real-time data,” writes Townsend (2014), “which can lead to a significant reduction in both congestion and the environmental impact of urban transport”. As AI technology continues to advance, its role in traffic management will likely expand, enabling even more efficient and intelligent urban mobility solutions.
Traffic congestion not only impacts travel times but also contributes to air pollution and carbon emissions. Real-time traffic management systems help mitigate these environmental effects by optimizing traffic flow and reducing idling times at intersections. In addition, urban data platforms can incorporate environmental data, such as air quality sensors, to adjust traffic management strategies based on current conditions. According to Nieuwenhuijsen and Khreis (2019), “Reducing traffic congestion through real-time management leads to lower emissions of CO2 and other pollutants, which is essential for cities striving to meet their sustainability goals”. This highlights the dual benefit of such systems in improving both urban mobility and environmental quality.
Despite their advantages, the implementation of real-time traffic management systems through urban data platforms is not without challenges.
“While urban data platforms offer significant benefits, their implementation requires careful planning to address challenges related to cost, privacy, and system integration,” Allam and Newman (2018).
The future of real-time traffic management is closely tied to the rise of autonomous vehicles and smart infrastructure. Autonomous vehicles (AVs) generate massive amounts of data that can be fed into urban data platforms, allowing for more precise traffic management. Furthermore, smart infrastructure, such as connected traffic lights and road sensors, will play a crucial role in ensuring the smooth operation of AVs within city environments.
As Gehl (2018) writes, “The development of smart infrastructure, combined with the proliferation of autonomous vehicles, will necessitate a new approach to urban traffic management, one that relies heavily on real-time data and predictive analytics”. This signals a future where cities are not just reacting to traffic conditions but actively shaping mobility patterns through intelligent, connected systems.
Real-time traffic management solutions powered by urban data platforms represent a significant advancement in addressing the complexities of urban mobility. By leveraging real-time data, predictive analytics, and AI technologies, cities can optimize traffic flow, enhance safety, reduce environmental impacts, and improve the overall quality of life for residents.
While challenges exist in implementing these systems—such as high costs, technical complexities, and data privacy concerns—the benefits are substantial. Successful adoption requires collaboration among government agencies, technology providers, researchers, and the public.
Looking ahead, the emergence of autonomous vehicles and smart infrastructure will further transform traffic management. Urban data platforms will become increasingly important in coordinating these technologies, enabling cities to move toward more sustainable, efficient, and intelligent transportation systems.
As urbanization continues and the demands on transportation networks grow, embracing real-time traffic management solutions will be essential for cities seeking to thrive in the 21st century.
Minza Shahid is a student, ardent about urban design and enhancing public spaces and aims to utilize architecture to foster community and sustainable environments. She is also a writer who explores the cultural and anthropological elements of the transforming built environment.
Visualising Urban and Architecture Diagrams
Session Dates
“Let’s explore the new avenues of Urban environment together “
Cookie | Duration | Description |
---|---|---|
cookielawinfo-checkbox-functional | 11 months | The cookie is set by GDPR cookie consent to record the user consent for the cookies in the category "Functional". |
viewed_cookie_policy | 11 months | The cookie is set by the GDPR Cookie Consent plugin and is used to store whether or not user has consented to the use of cookies. It does not store any personal data. |
cookielawinfo-checkbox-analytics | 11 months | This cookie is set by GDPR Cookie Consent plugin. The cookie is used to store the user consent for the cookies in the category "Analytics". |
cookielawinfo-checkbox-performance | 11 months | This cookie is set by GDPR Cookie Consent plugin. The cookie is used to store the user consent for the cookies in the category "Performance". |
cookielawinfo-checkbox-necessary | 11 months | This cookie is set by GDPR Cookie Consent plugin. The cookies is used to store the user consent for the cookies in the category "Necessary". |
cookielawinfo-checkbox-others | 11 months | This cookie is set by GDPR Cookie Consent plugin. The cookie is used to store the user consent for the cookies in the category "Other. |
One Comment